Visualizing the gradient descent method
Por um escritor misterioso
Descrição
In the gradient descent method of optimization, a hypothesis function, $h_\boldsymbol{\theta}(x)$, is fitted to a data set, $(x^{(i)}, y^{(i)})$ ($i=1,2,\cdots,m$) by minimizing an associated cost function, $J(\boldsymbol{\theta})$ in terms of the parameters $\boldsymbol\theta = \theta_0, \theta_1, \cdots$. The cost function describes how closely the hypothesis fits the data for a given choice of $\boldsymbol \theta$.
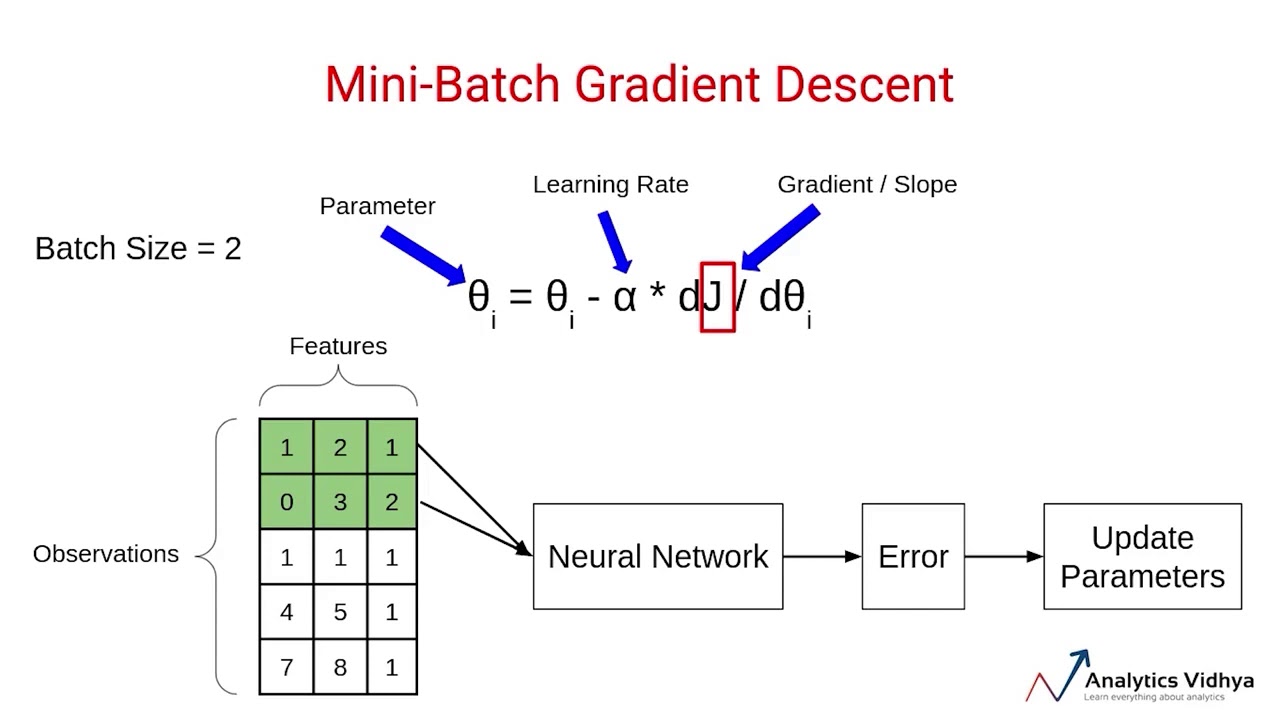
Variants of Gradient Descent Algorithm
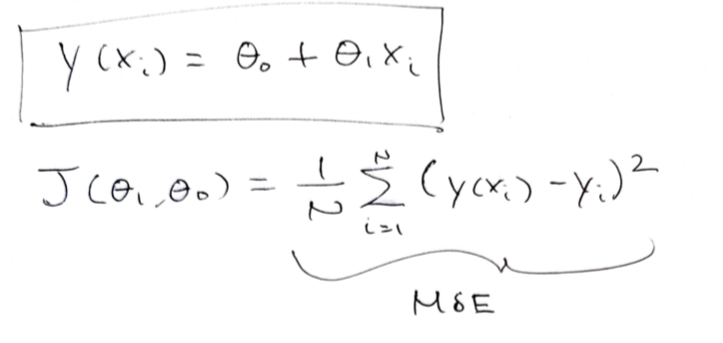
Visualizing the gradient descent in R · Snow of London
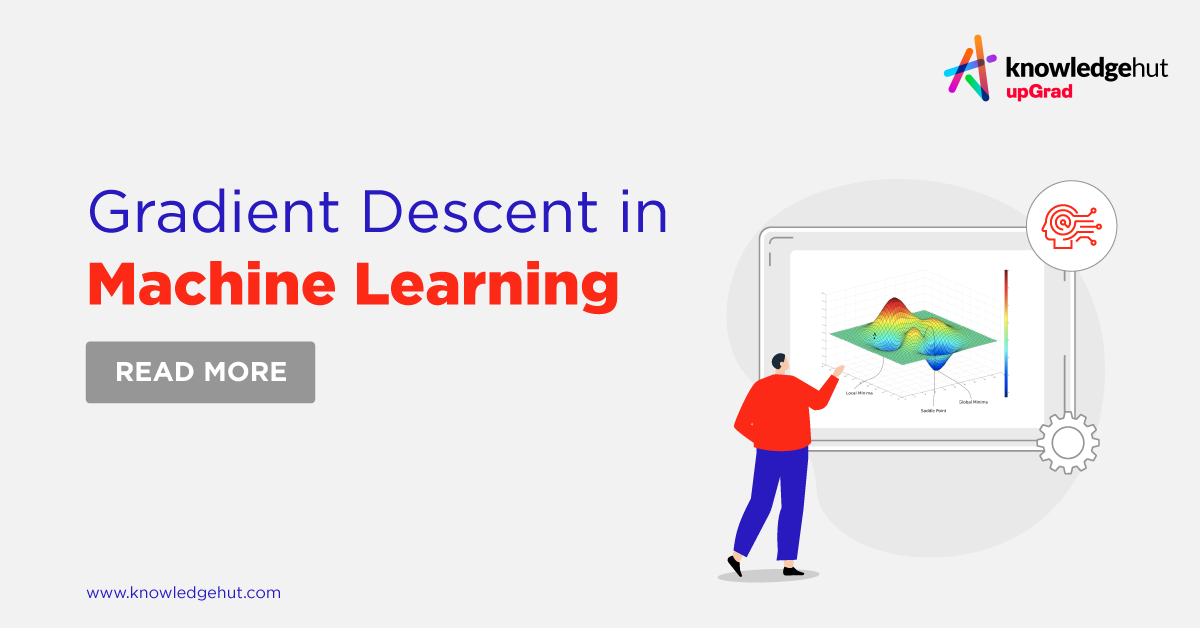
Gradient Descent in Machine Learning: What & How Does It Work
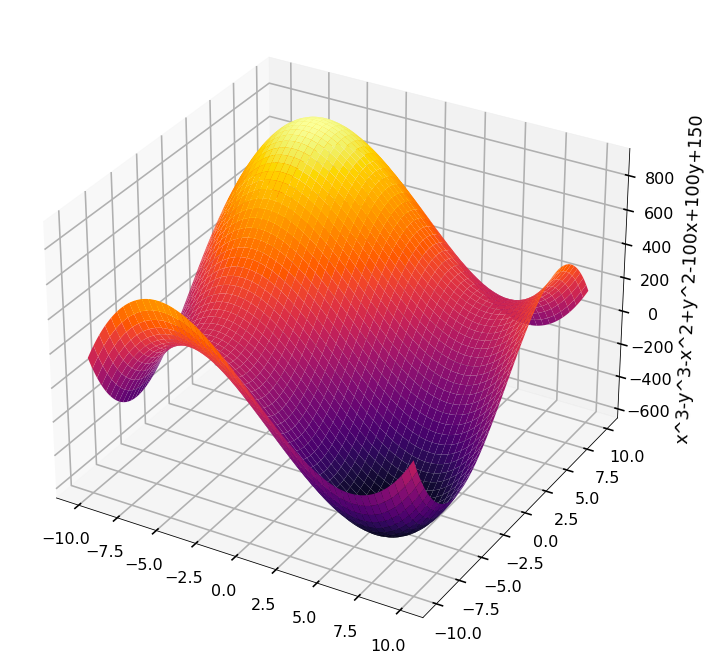
Gradient Descent Visualization - Martin Kondor

Gradient Descent vs Adagrad vs Momentum in TensorFlow
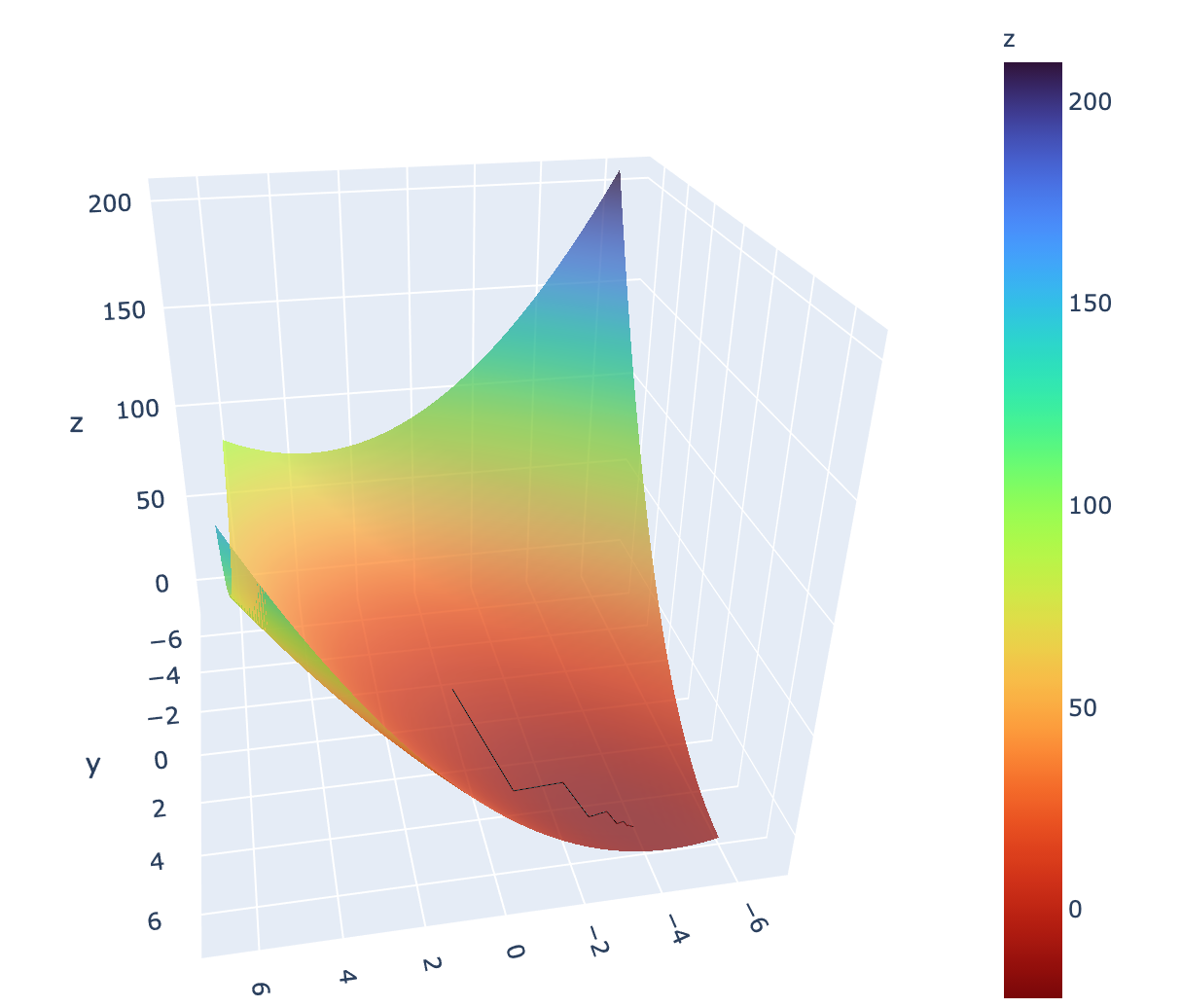
Descent method — Steepest descent and conjugate gradient in Python, by Sophia Yang, Ph.D.
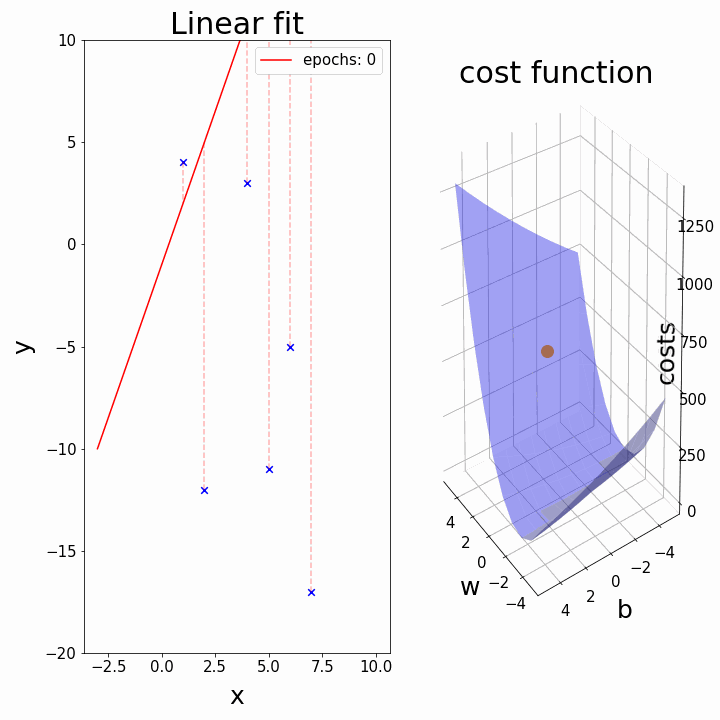
Gradient Descent animation: 1. Simple linear Regression, by Tobias Roeschl

How to visualize Gradient Descent using Contour plot in Python
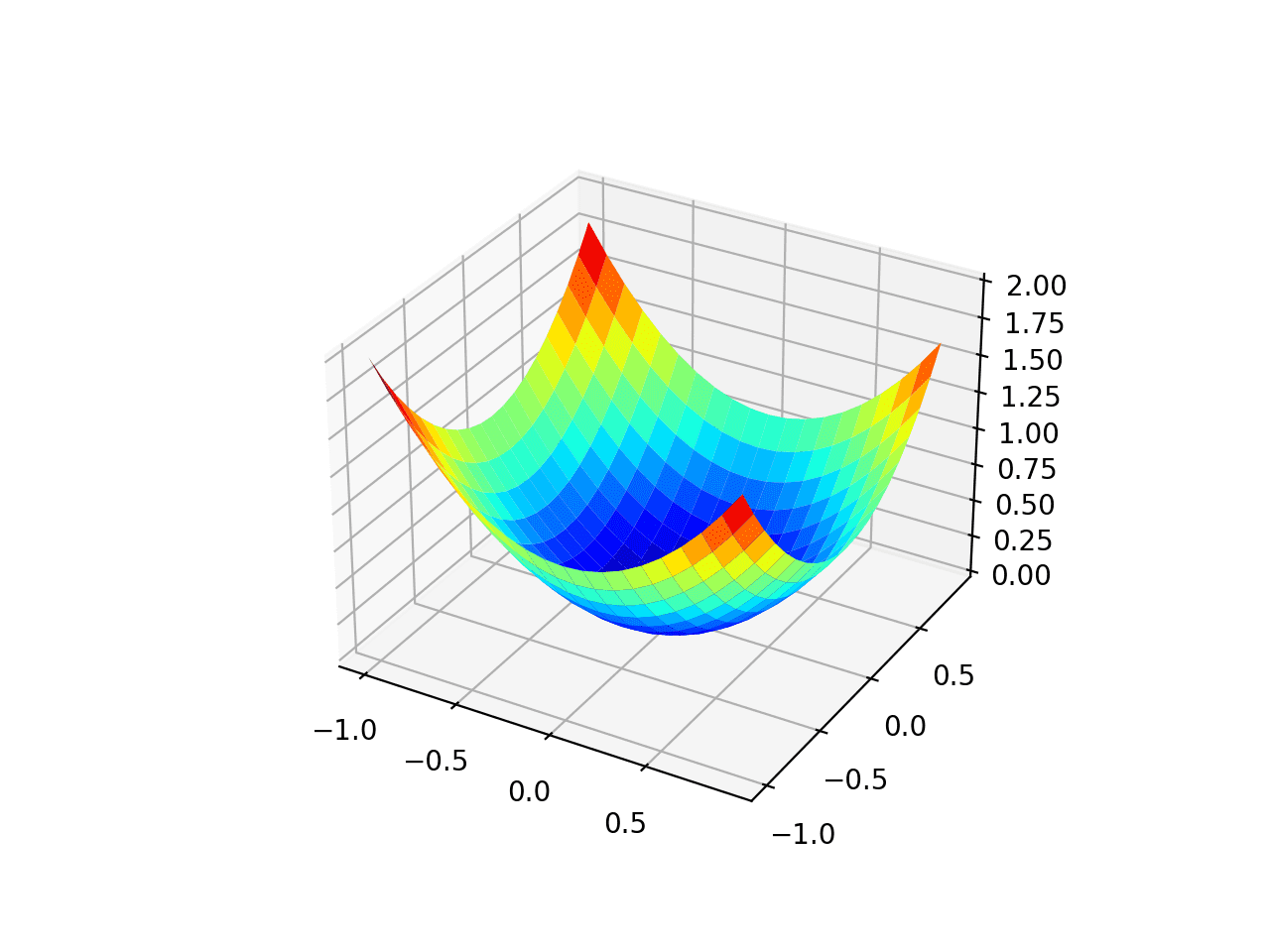
Gradient Descent With AdaGrad From Scratch
Gradient Descent from scratch and visualization
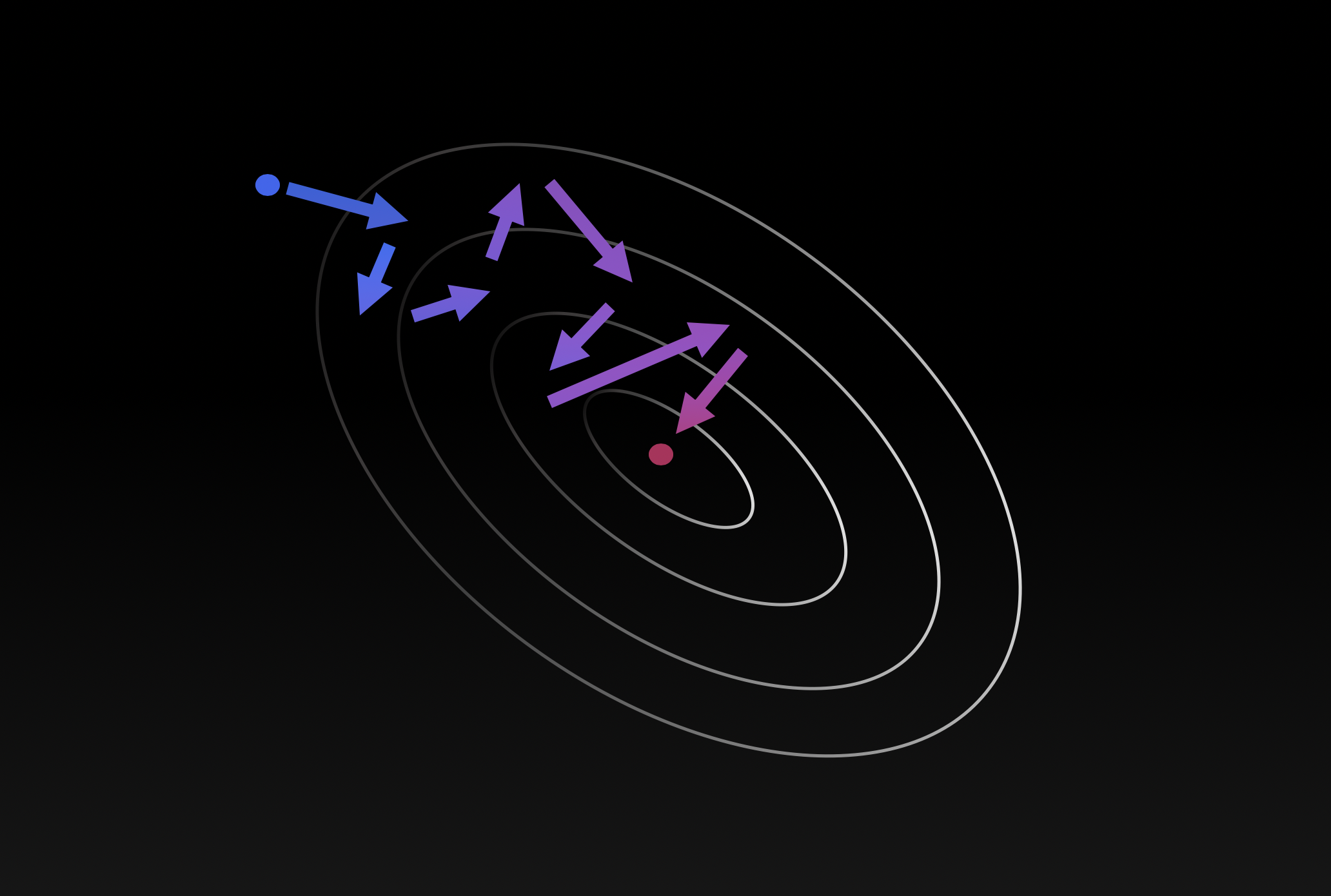
ZO-AdaMM: Derivative-free optimization for black-box problems - MIT-IBM Watson AI Lab