Pharmaceutics, Free Full-Text
Por um escritor misterioso
Descrição
Exposure-response (E-R) is a key aspect of pharmacometrics analysis that supports drug dose selection. Currently, there is a lack of understanding of the technical considerations necessary for drawing unbiased estimates from data. Due to recent advances in machine learning (ML) explainability methods, ML has garnered significant interest for causal inference. To this end, we used simulated datasets with known E-R “ground truth” to generate a set of good practices for the development of ML models required to avoid introducing biases when performing causal inference. These practices include the use of causal diagrams to enable the careful consideration of model variables by which to obtain desired E-R relationship insights, keeping a strict separation of data for model-training and for inference generation to avoid biases, hyperparameter tuning to improve the reliability of models, and estimating proper confidence intervals around inferences using a bootstrap sampling with replacement strategy. We computationally confirm the benefits of the proposed ML workflow by using a simulated dataset with nonlinear and non-monotonic exposure–response relationships.

Pharmacy Technician - CVS
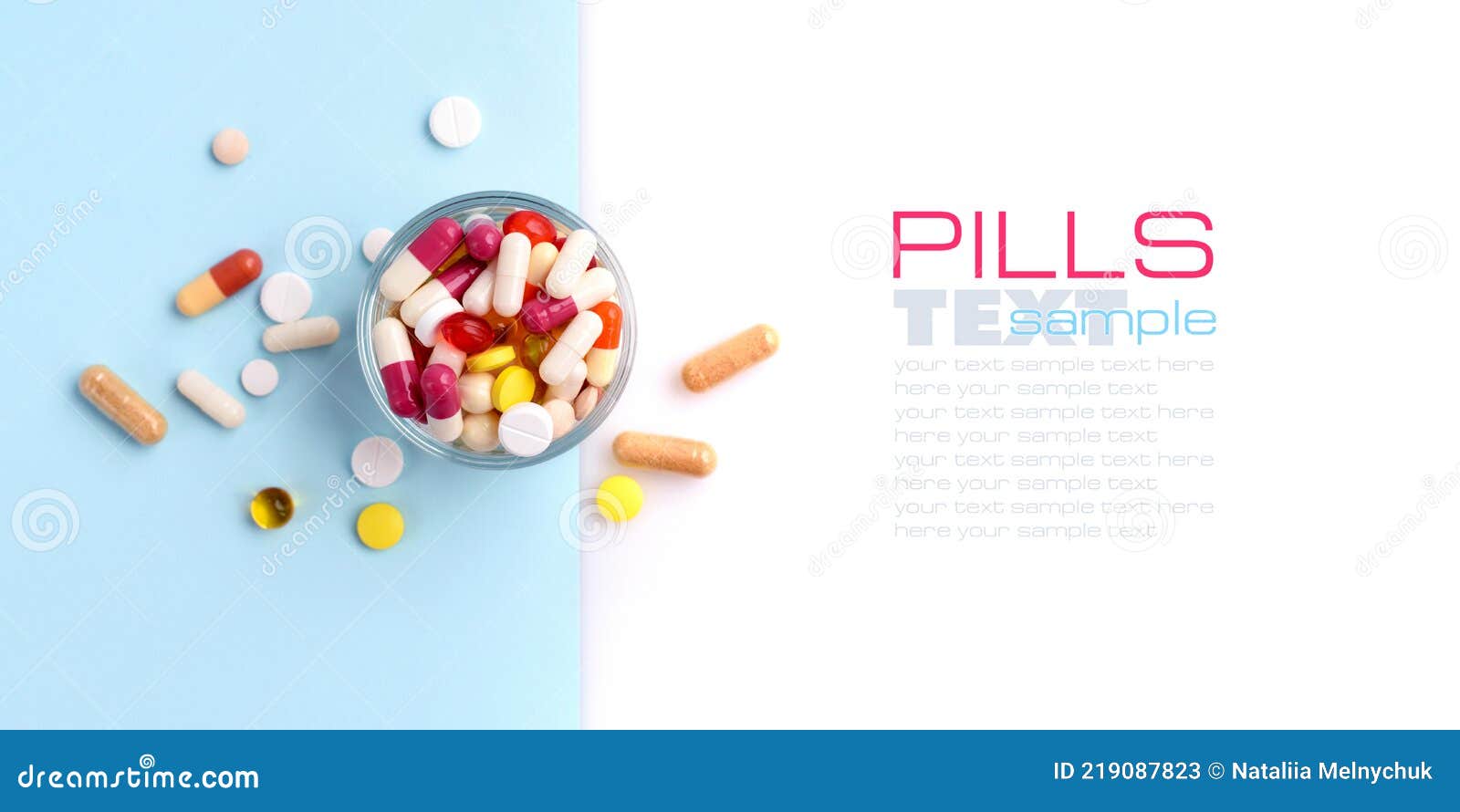
115 Empty Pharma Capsules Stock Photos - Free & Royalty-Free Stock
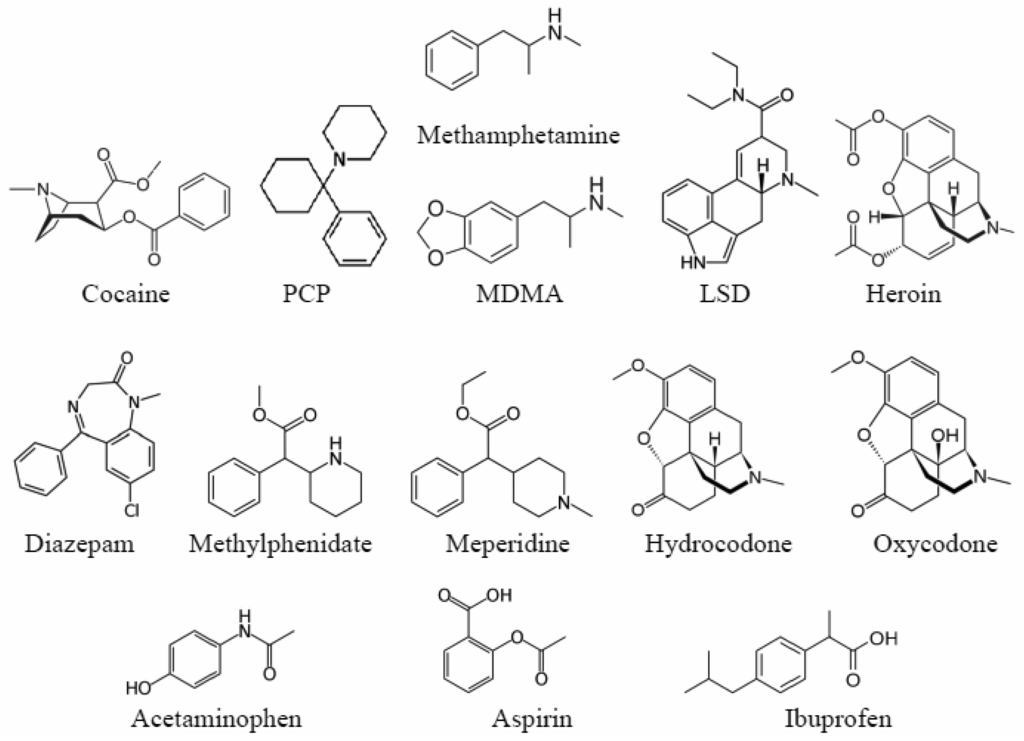
Pharmaceutics, Free Full-Text
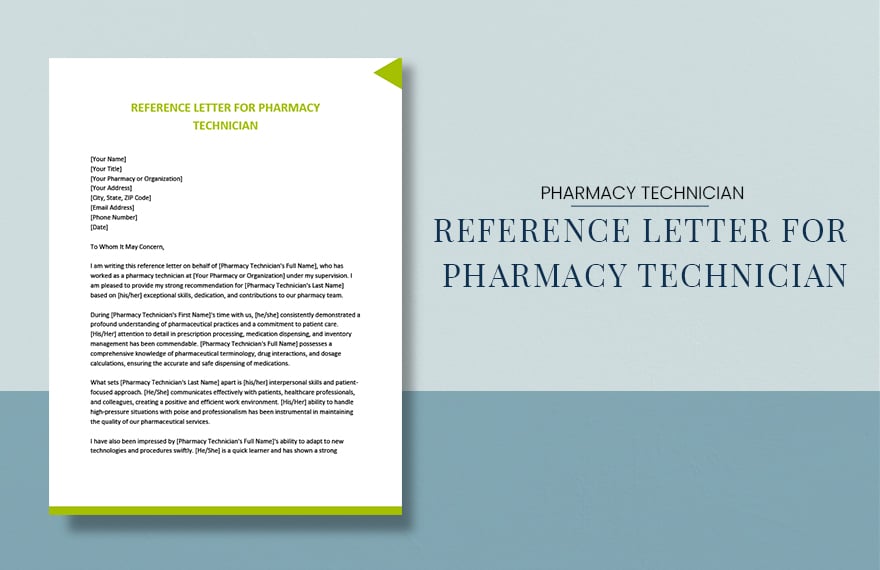
Free Reference Letter For Pharmacy Technician - Download in Word
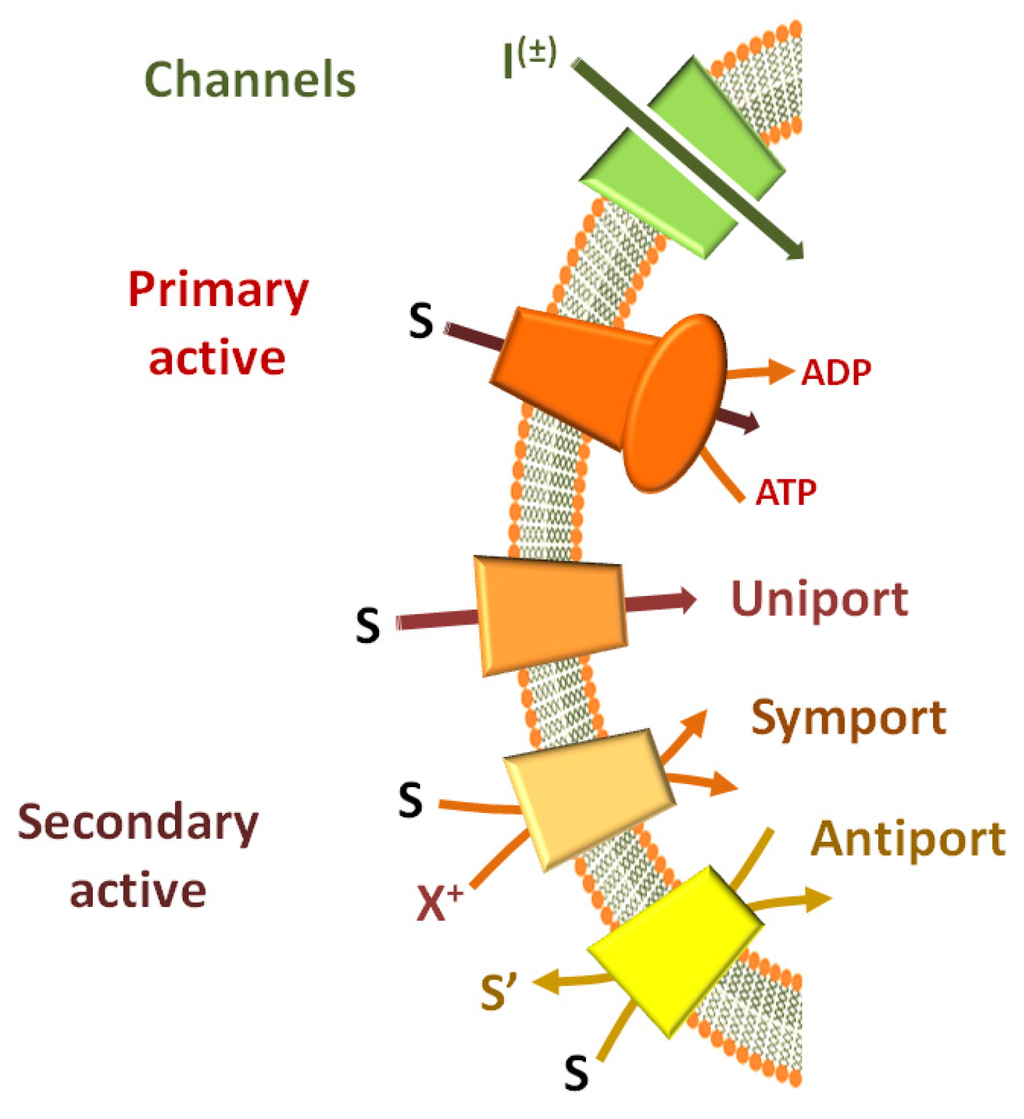
Pharmaceutics, Free Full-Text
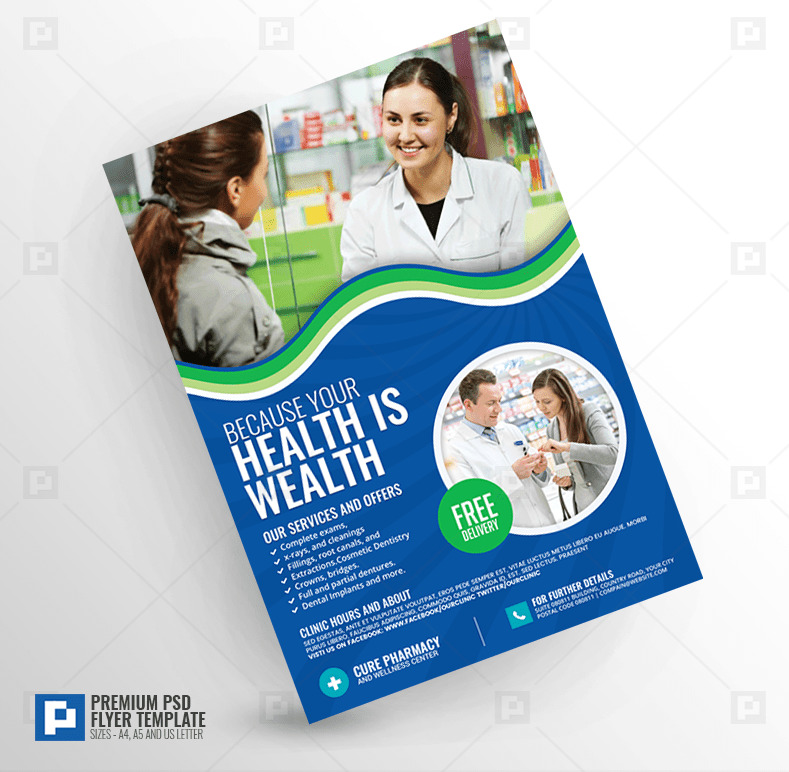
Pharmaceutical Services Flyer - PSDPixel

Free Download Pharmaceutical Product Strategy by angeliqblue - Issuu
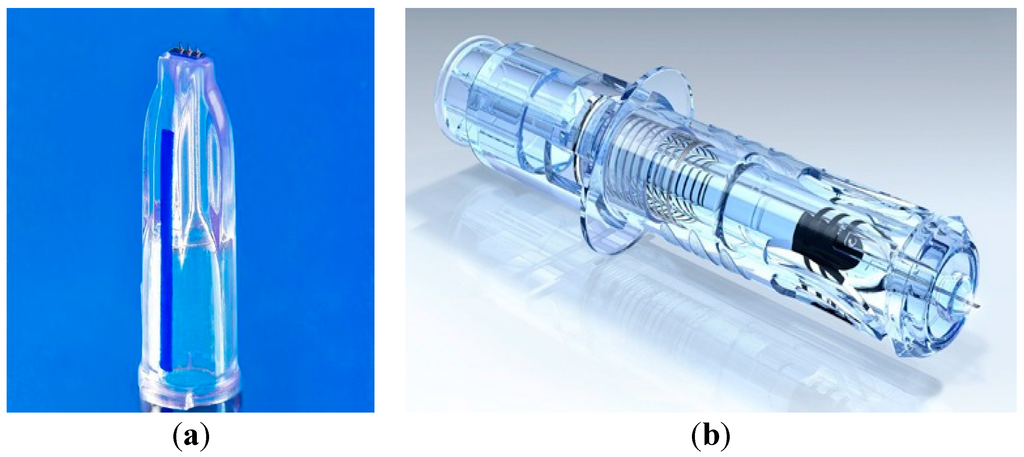
Pharmaceutics, Free Full-Text
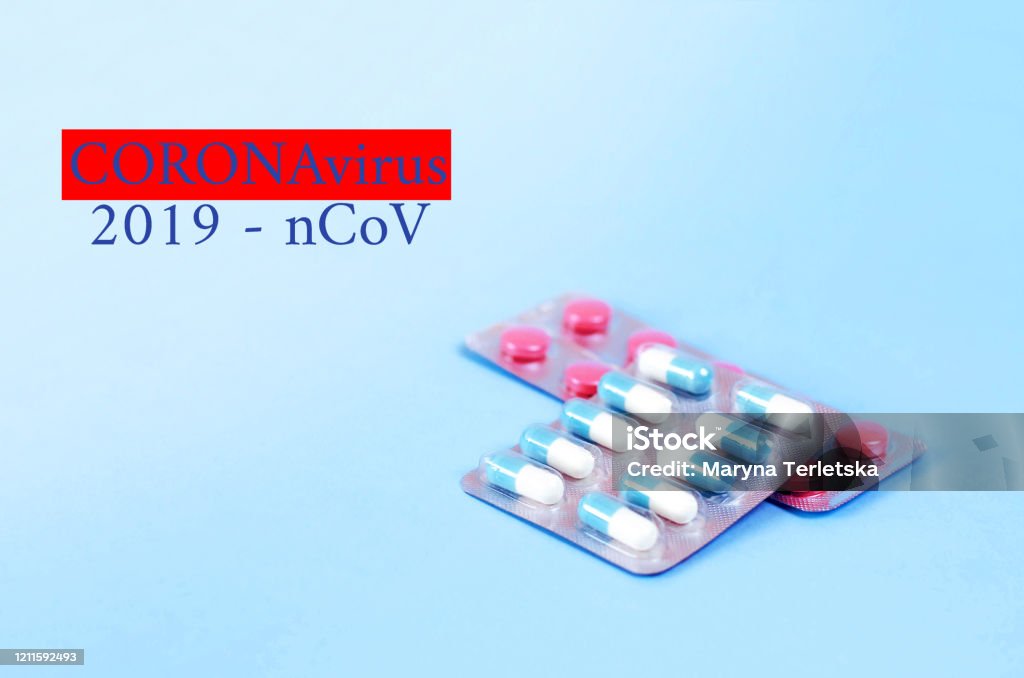
Text With The Full Name Of Coronaris Disease And Pills Stock Photo