Machine Learning Chapter 7. Computational Learning Theory Tom M. Mitchell. - ppt download
Por um escritor misterioso
Descrição
3 Computational Learning Theory (2/2) What general laws constrain inductive learning? We seek theory to relate: –Probability of successful learning –Number of training examples –Complexity of hypothesis space –Accuracy to which target concept is approximated –Manner in which training examples presented
(maximum over all possible c C, and all possible training sequences) Definition: Let C be an arbitrary non-empty concept class. The optimal mistake bound for C, denoted Opt(C), is the minimum over all possible learning algorithms A of M A (C)..
(maximum over all possible c C, and all possible training sequences) Definition: Let C be an arbitrary non-empty concept class. The optimal mistake bound for C, denoted Opt(C), is the minimum over all possible learning algorithms A of M A (C)..
Machine Learning in QM/MM Molecular Dynamics Simulations of
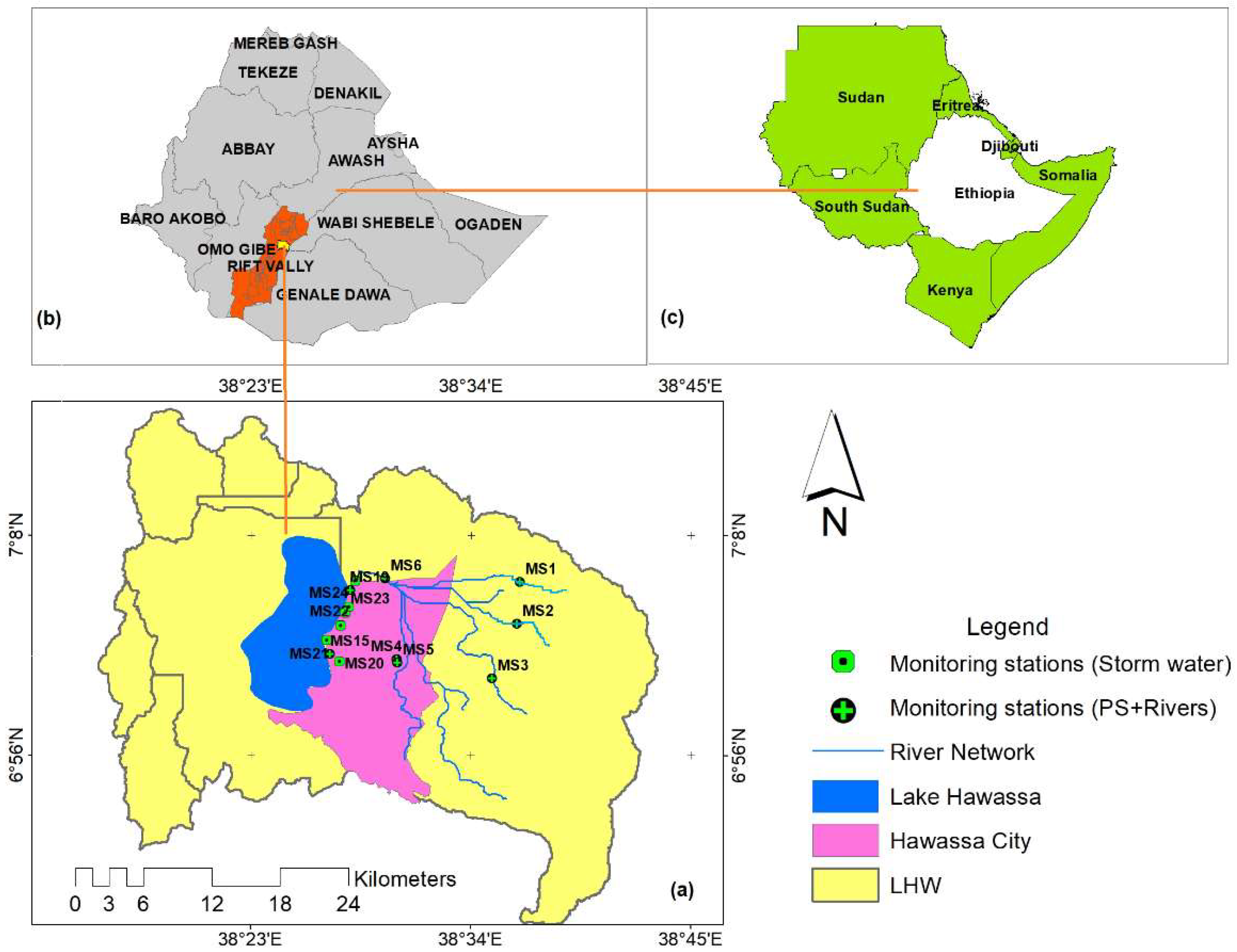
Water, Free Full-Text

RNA-binding proteins balance brain function in health and disease

Behavior of tropopause height and atmospheric temperature in
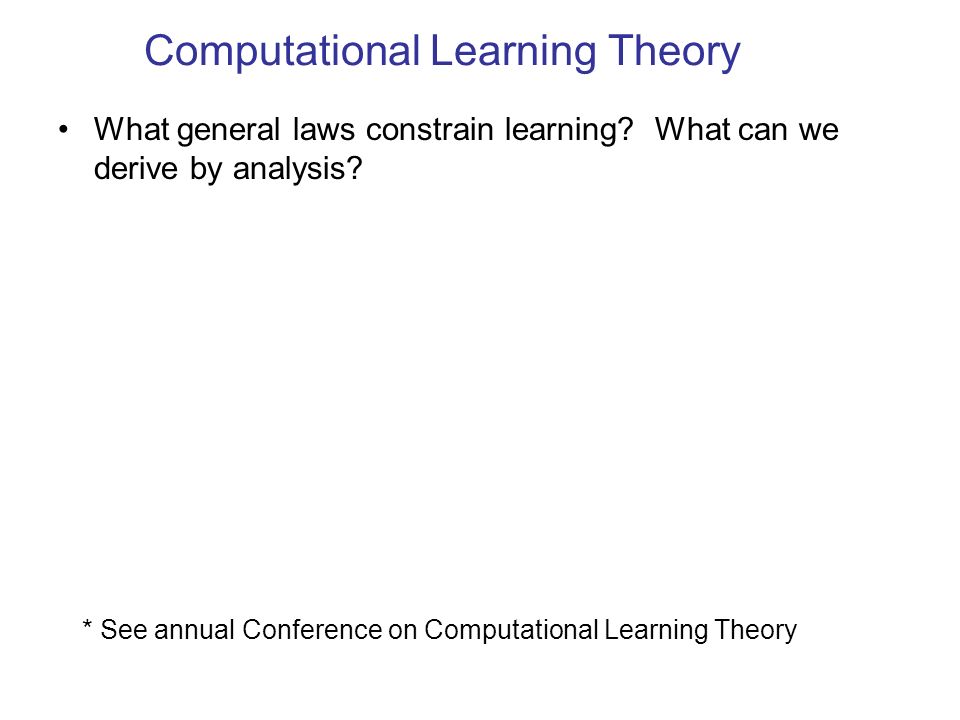
Machine Learning Tom M. Mitchell Machine Learning Department
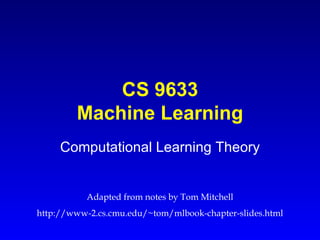
Computational Learning Theory

Machine Learning 3 - Decision Tree Learning
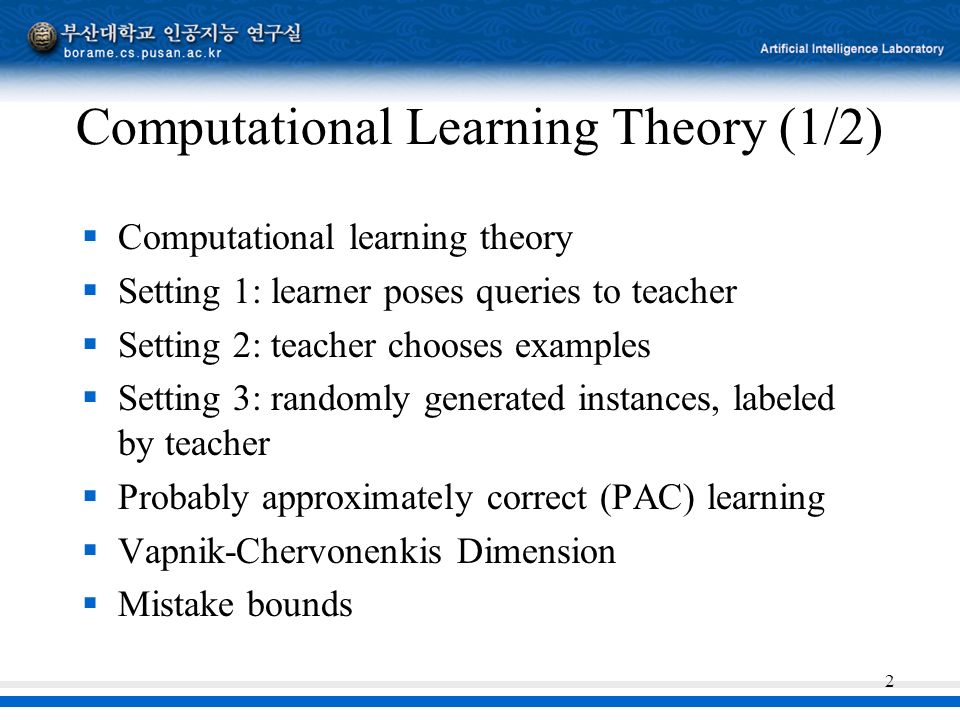
Machine Learning Chapter 7. Computational Learning Theory Tom M

Machine Learning : Mitchell, Tom M. (Tom Michael), 1951- author
Machine Learning (15Cs73) : Text Book Tom M. Mitchell, Machine
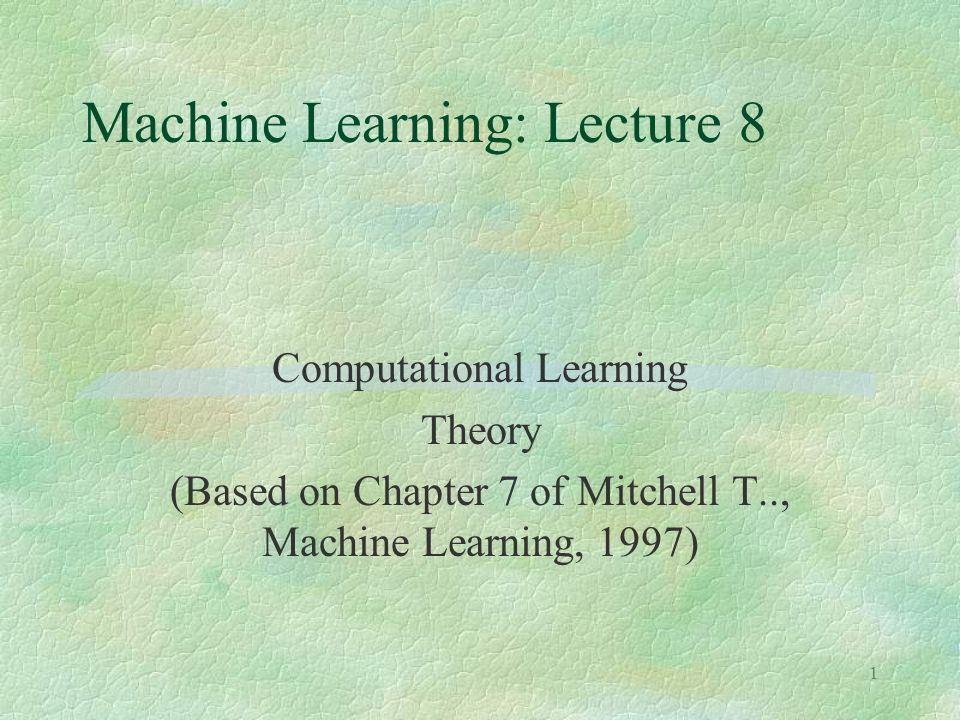
1 Machine Learning: Lecture 8 Computational Learning Theory (Based
The International Science and Evidence-based Education Assessment

Challenges in Deploying Machine Learning: A Survey of Case Studies
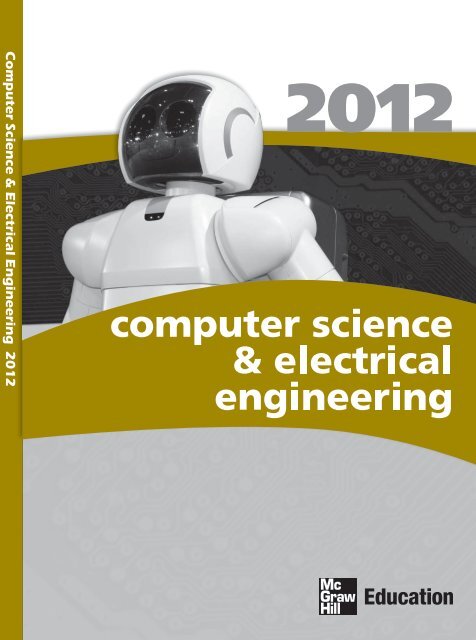
Computer Science & Electrical Engineering 2012 - McGraw-Hill Books